

Fingerprinting the Brain
research
​
​Abstract: EEG-Based Neural Biometrics:
Fingerprinting the Human Brain Research Project
​
Introduction
Secure, reliable methods for individual identification are increasingly vital in high-security domains such as defense, healthcare, and cybersecurity. Traditional biometric systems—like fingerprints and facial recognition—are increasingly susceptible to spoofing and replication. In pursuit of a more secure alternative, researchers at PVNet launched an independent study to explore whether electroencephalography (EEG) data could serve as a biometric identifier. This effort was inspired by a parallel Department of Defense-funded initiative awarded to IEEE scientists.
Methodology
Test Environment:
To minimize sensory interference, a blackout room was constructed to serve as the test chamber. Subjects were fitted with Emotiv+ EEG headsets, which provide high-resolution brainwave recordings in an accessible format.
Stimuli Design & Data Acquisition:
Participants viewed a controlled series of visual stimuli—images and short video clips—while engaging in light cognitive tasks. The presentation was managed via custom-developed software to ensure consistent timing and order across all 10 test subjects.
Signal Processing:
EEG data was preprocessed to remove common artifacts such as muscle movement and eye blinks. Signals were normalized to enable valid comparisons between individuals and across sessions.
Feature Extraction & Pattern Identification:
Custom MATLAB routines were developed to extract defining features from the EEG signals. Statistical analysis was used to identify subject-specific neural signatures. The testing process was iteratively refined by determining which stimuli best elicited unique and consistent responses.
​
Profile Generation and Reverse Testing:
The system generated neural profiles for each participant, capturing the unique characteristics of their EEG activity. To evaluate the accuracy and robustness of these profiles, reverse testing was conducted. In this phase, previously recorded visual stimuli were randomly presented again to participants. EEG data was captured in real-time and analyzed using the custom MATLAB software to match the incoming signals against the existing database of neural profiles—testing whether the system could correctly identify the subject based solely on their brainwave responses.
​
Results
Preliminary findings indicate that EEG-based identification is both viable and promising. Participants exhibited neural signatures that were consistent across trials and distinct from one another. During reverse testing, the system was able to match real-time EEG data to the correct pre-recorded profile with high accuracy, further validating the potential of “brain fingerprinting” as a new class of biometric.
​
Conclusion
This research supports the feasibility of using EEG signals for secure identity authentication. Compared to traditional biometrics, brainwave patterns offer deeper intrinsic security due to their complexity and resistance to imitation. As EEG hardware, machine learning, and neuroinformatics continue to evolve, brain-based biometric systems may play an essential role in future identity management. Beyond the technical outcomes, this project offered PVNet interns meaningful exposure to neuroscience, software development, and biometric systems—demonstrating the dual value of innovation and STEM education.
​​​​
​​​​​
​​
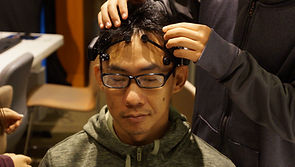
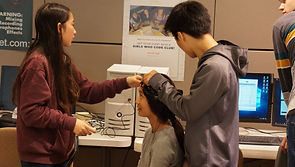

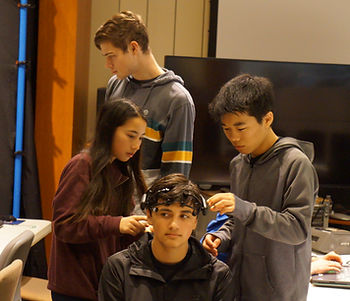

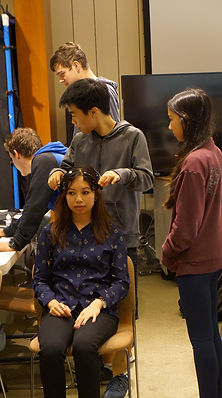

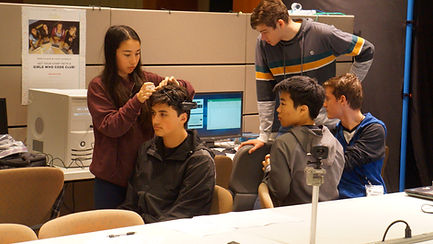
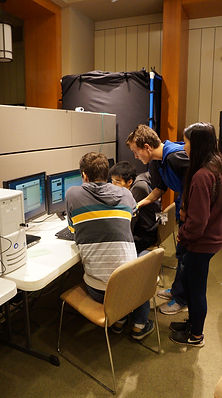

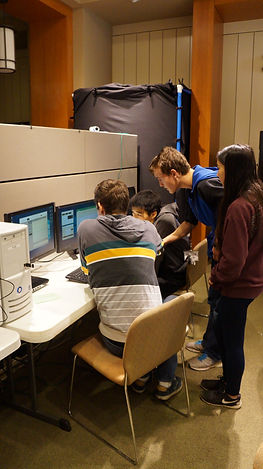
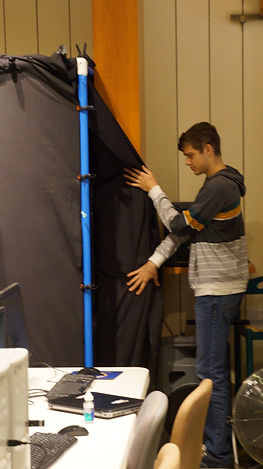

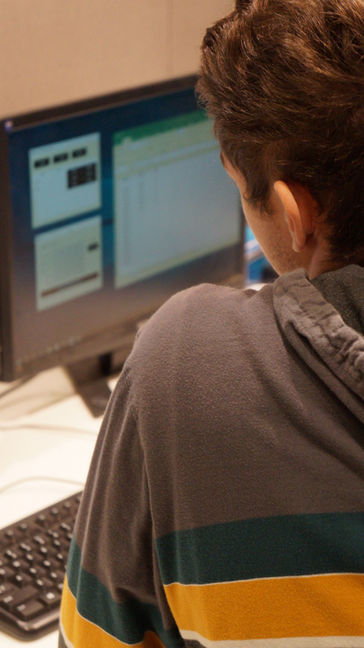
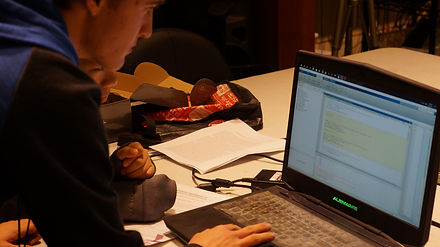
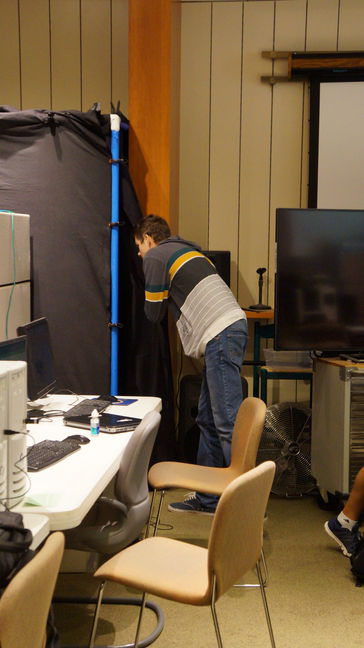
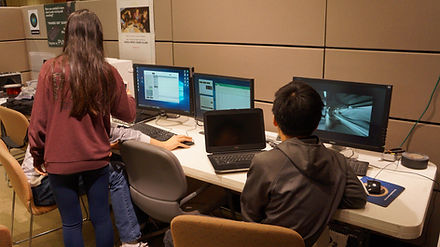
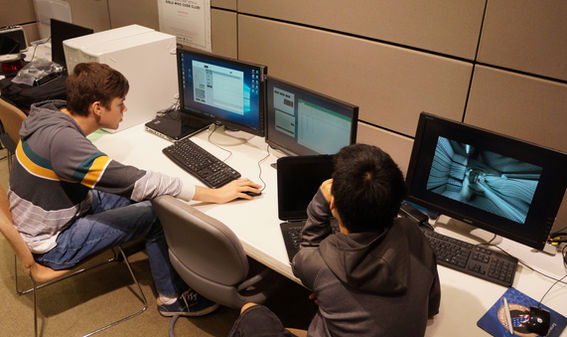
